Complex Systems Summer School 2011-Blog: Difference between revisions
From Santa Fe Institute Events Wiki
No edit summary |
No edit summary |
||
(10 intermediate revisions by 2 users not shown) | |||
Line 1: | Line 1: | ||
{{Complex Systems Summer School 2011}} | {{Complex Systems Summer School 2011}} | ||
Use this page as an informal forum to share your opinion and discuss anything at CSSS' | Use this page as an informal forum to share your opinion and discuss anything at CSSS'11. | ||
Students are encouraged to share their observations, insights, and opinions about daily lecture content as well as extracurricular activities. | Students are encouraged to share their observations, insights, and opinions about daily lecture content as well as extracurricular activities. | ||
Students may be assigned a day to provide a free-form blog entry covering the topics of that day. | Students may be assigned a day to provide a free-form blog entry covering the topics of that day. | ||
== a great, hilarious and informative documentary on "the new science of networks" == | |||
Or at least, that is what a collegue of mine said about this documentary. | |||
I haven't seen it yet, but is sounds interesting... | |||
[http://www.youtube.com/watch?v=RcCpEf6_Ofg Link to documentary on new science of networks] | |||
== Does a picture blog count, too? == | |||
All my pictures so far can be found at | |||
[https://picasaweb.google.com/101826271245347003012/SantaFe?authkey=Gv1sRgCOLn6fC9v-aR1AE this website] <br> | |||
I have trouble choosing between pictures, so I just put almost everything up there. The cat, the hike, some summer-school-in-action, and much more. | |||
I have to say, I should actually tweak around with the colors and stuff, but there is simply no time. | |||
Feel free to send me an email what your favorite picture is (nr + description), and I will send you the raw file with which you can tweak around yourself! | |||
Best, | |||
Evelien | |||
[[File:Cat.jpg|left|440px|alt=Cat at SFI]]<br> | |||
== What is Complexity? - a first attempt to explain it to 'my grandmother', by Evelien == | |||
After 2.5 weeks of complex systems summerschool, it might be time to tell about what complexity is. You might think this is an easy task after so many back to back lectures, but this is not the case. A good example is the favorite definition of complexity uttered here that stuck to the mind of most (guys): | |||
''Complexity is like pornography: you know it when you see it.'' | |||
You can say a lot about this definition, but I think we can all agree that this definition does not really help you to understand what complexity is. I hope to do better on that account, by sketching the broad line I noticed in the lectures so far. It is important though to note that clearly from the above definition, even professionals in this field do not have one clear concept of what a complex system is. So, if after reading this (rather long) piece you have a feeling of what a complex system might be – you are almost an expert ;) | |||
'''The general idea of Complexity''' | |||
You come across complexity and complex systems (I will interchange the terms freely) in many areas of science. The current participants illustrate that: we have computer scientists, social scientists, archeologists, geographical information systems-persons, Mathematicians, Physicists, Economists, and much more. I would say that the explanation for this diversity is that a complex system is not a well-defined thing or process, but rather a way of looking at things, and doing research. | |||
Some of these fields I mentioned above are ‘old’ sciences. People like Newton studied the world to try to understand it. Therefore, they made simplifying assumptions in order to study the workings of the world, or better: to make models of it. Next generations started too built on their research, then the next generation built on that research, new fields started to emerge until we have the broad science field of today. | |||
Fortunately, we have made some progress in the past few decades or even centuries. This allows us to go far, far back to the core models of the field, and question what assumptions were made in that model – and whether we think this is a viable representation of the real world. | |||
A good complex systems scientist will always be able to find something which is important – but currently left out. An even better complex systems scientist can borrow from other fields of research and using that knowledge to extend the model to include a new, more complicated assumption, which will make the whole process more complex. And that basically is a complex system. | |||
'''Examples of Complex Systems''' | |||
Not every extension of a model will make it directly a complex system: the problem itself needs to become fundamentally harder. To keep you some feeling of complex systems, I will describe three examples. | |||
''Independence assumption'' | |||
Especially in statistics, and ‘identically independent distributed process’ is one of the favorite toys of scientists. The independency assumption basically means that when two things happen, they do not depend upon each other. Take for instance the waiting line at the supermarket: people are assumed to be joining at random. However, when the waiting line is very long, you might decide that you need to shop faster and join sooner (because you need to make an appointment), then when the line is short. So, the process may not be independent. What happens in the case of dependency is what Jim Crutchfield talked about today (all day, and still not finished ;) ). | |||
''Continuity assumption'' | |||
Both in math and in physics, often it is assumed that you can approximate a discrete process by a continuous process. Some of you may remember the Riemann-sums (blocks underneath the graph) to approximate the area underneath the graph. The next step was to integrate the function, and use that to calculate the area underneath the graph. The latter (integrated function) is the continuous process version of the discrete process (the Riemann-sum). | |||
Once you have integrated the function, calculating the area underneath a graph is easier than with the Riemann-sum – especially when you want to do more advanced stuff. That is why often people approximate a discrete process by a continuous process: as it generally makes things easier, and allows for exact mathematical results. But, a continuous process is not always a good representation of a discrete process. | |||
A counter example turns out to be childishly simple (and fun). Remember the little water/sand mills for children? You put in a substance on the top, and as soon as the first bucket is full, the wheel starts to turn. If you have a continuous flow of e.g. water, the wheel will keep turning – and in this case a continuous process is a good approximation to find e.g. the speed of the wheel. | |||
But, when we take the same wheel, and we slowly start dripping water into it, something different happens. At some frequency (and you can try this yourself) the little bucket will drip full slowly, and then turn a bit as it loses balance. A new little bucket is up, and that will drip full – but may start turning the wheel in a different direction then the one before. Predicting which way the wheel will turn, becomes a very difficult task – which issue would never be clear from the continuous process. So, the discrete process shows a feature that we cannot capture in the continuous representation. | |||
Off course, the way a child’s toy turns seems not vital to the working of the world at large. But in fact, this is a small model of how a discrete process can result in behavior that is different from the continuous approximation – and that discovery (and playing with that) is complexity science, too. | |||
''Networks'' | |||
Finally, I couldn’t stop before I mentioned networks. In short, this is the field of studying interactions and relations between all kinds of things: people, the internet, power grids, and so on. Basically, the network is studied in terms of connectedness, robustness, distance between nodes, and much more. Secondly, they link these things to research fields in which networks are important, such as transportation, but also human interaction. In the spread of (contagious) disease, the network of people connecting to one another is a vital part of the way disease spreads. So, studying epidemic outbreaks as a complexity scientists, you would include the (social)networks of the people involved in finding the best way to fight the disease. | |||
''''New techniques'''' | |||
Generally, complex systems are at the front end of their research field (or, on the far boarder of their fields, if you are a bit more skeptical). You need some guts when you decide to study the internet as an organism. You need to have a dream if you are working on building a super computer with far more power than the human brain. And you may be considered plainly stupid by some, if you spent your time programming little agents who interact with each other. In short, as a complexity scientist you need to be confident and believe that you will bring science to the next level. | |||
These complex systems are complex because they cannot be easily solved by standard technology of the field, new methods need to be developed – or can be developed using complex systems. A good example of the latter is that Alfred Huebler can let small iron bits play tetris (but as mentioned before, Alfred Huebler is said to be able to do anything if he just ‘puts up the throughput’- including wrestling crocodiles). An example of the former (new methodology) is the ever growing attention for agent based modeling: in the computer program Netlogo this would be equivalent to programming how turtles interact with each other: basically making a ‘game’ in which you tell every single turtle to e.g. turn around when he sees land, and go towards the nearest turtle he can see. All of that is complex systems research. | |||
'''Why should you be happy there are ‘complex systems scientists’?''' | |||
In case you are a scientist yourself, this is pretty easy: complexity research is a lot of fun, you can do very different things, meet people from many different fields, it allows for an open mind, creativity and challenging problems – and on top of that, it is very much in fashion in the greater scientific community, which may benefit your chance of getting something published. | |||
Chances are, you are not a scientist. Why should you care? Computers taking over the world doesn’t sound like a good thing to invest taxes in, I could imagine. I am not going to completely contradict that notion: it is true that some of this research is pretty wild, and ‘we’ are not sure if ever anything useful will come out. I do not know if this is a consolation, but that is unfortunately true for a lot of research (if not all). | |||
There is an upside: complexity research so far has lead to some pretty cool results! When trying to prevent an epidemic (large spread of disease) I think it makes sense to take into account that a busdriver will meet far more people that the average ‘I prefer to work at home’ scientist – and taking that into account in to the modelling may make the way we fight spread of disease far more effective. | |||
Another example I find pretty cool, is that someone has made an agent-based model of a long-gone cultural society. Archeologists are better able to reconstruct and explain/clarify the way the people lived. For instance, they find that after so many years food starts to get scars in the area, so the import chicken to keep near their houses, so that they have enough to eat. | |||
The same agent based models can explain some of the stuff currently going on in our society: from segregation of ethnicities to marriage… So, although we are not sure all complexity research is of benefit to our world, there are some pretty neat examples that say complexity research makes the world a little bit a better place. | |||
'''In summary''' | |||
In essence, complexity goes back to the roots of the model and the problem, compares this to the world, finds an important feature that is still missing from the model, and tries to add this. Additions can be e.g. interactions between things in the model, nonlinearity (not everything is a straight line), dependency of things (not all processes are purely random), and so on. | |||
To solve these problems, complexity researchers try to borrow from many fields, but two of their favorites are statistics and mathematics. Another option instead of borrowing is to develop new techniques to study (new) problems. | |||
Not all complexity research is immediately clearly usefull. Still, there are good examples of where complexity research has made a huge difference in the way we understand or solve things, such as understanding and accommodating for interactions in a process (think of the disease spread and network). | |||
Complexity researchers come from all different kinds of backgrounds and fields – and this probably causes the fact that there is no single, clear definition of a complex system. It might even be (in my opinion), that the current ‘new’ assumptions of complexity will get accepted in each of their respective fields, and the in some years the current labeled ‘complex systems’ will not be considered to be complexity anymore, but continue their lives under a different name. | |||
I hope though, that the essence of this complexity field will not fade. In this highly fractured world, I think it is so very important to try to come together with people from different fields and with different specialties, learn from each other and be able to step back and compare our research to what we are actually studying: the place we live. I think this is vital to do good research and especially try to push research forward in a more converging instead of diverging way. | |||
Meeting different people and comparing your own believes and assumptions with the world, can be rewarding for everyone - scientist, and non scientist. Therefore I would like to end, now that you have a little bit of feeling for complexity, by wishing everyone a little complexity in their life. |
Latest revision as of 22:14, 28 June 2011
Complex Systems Summer School 2011 |
Use this page as an informal forum to share your opinion and discuss anything at CSSS'11.
Students are encouraged to share their observations, insights, and opinions about daily lecture content as well as extracurricular activities.
Students may be assigned a day to provide a free-form blog entry covering the topics of that day.
a great, hilarious and informative documentary on "the new science of networks"
Or at least, that is what a collegue of mine said about this documentary. I haven't seen it yet, but is sounds interesting... Link to documentary on new science of networks
Does a picture blog count, too?
All my pictures so far can be found at
this website
I have trouble choosing between pictures, so I just put almost everything up there. The cat, the hike, some summer-school-in-action, and much more.
I have to say, I should actually tweak around with the colors and stuff, but there is simply no time.
Feel free to send me an email what your favorite picture is (nr + description), and I will send you the raw file with which you can tweak around yourself!
Best,
Evelien
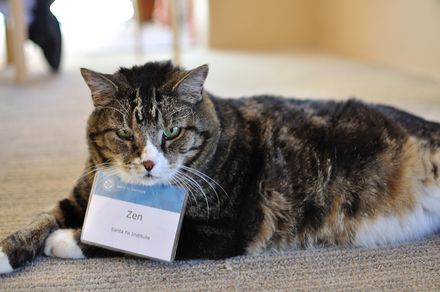
What is Complexity? - a first attempt to explain it to 'my grandmother', by Evelien
After 2.5 weeks of complex systems summerschool, it might be time to tell about what complexity is. You might think this is an easy task after so many back to back lectures, but this is not the case. A good example is the favorite definition of complexity uttered here that stuck to the mind of most (guys):
Complexity is like pornography: you know it when you see it.
You can say a lot about this definition, but I think we can all agree that this definition does not really help you to understand what complexity is. I hope to do better on that account, by sketching the broad line I noticed in the lectures so far. It is important though to note that clearly from the above definition, even professionals in this field do not have one clear concept of what a complex system is. So, if after reading this (rather long) piece you have a feeling of what a complex system might be – you are almost an expert ;)
The general idea of Complexity
You come across complexity and complex systems (I will interchange the terms freely) in many areas of science. The current participants illustrate that: we have computer scientists, social scientists, archeologists, geographical information systems-persons, Mathematicians, Physicists, Economists, and much more. I would say that the explanation for this diversity is that a complex system is not a well-defined thing or process, but rather a way of looking at things, and doing research.
Some of these fields I mentioned above are ‘old’ sciences. People like Newton studied the world to try to understand it. Therefore, they made simplifying assumptions in order to study the workings of the world, or better: to make models of it. Next generations started too built on their research, then the next generation built on that research, new fields started to emerge until we have the broad science field of today. Fortunately, we have made some progress in the past few decades or even centuries. This allows us to go far, far back to the core models of the field, and question what assumptions were made in that model – and whether we think this is a viable representation of the real world.
A good complex systems scientist will always be able to find something which is important – but currently left out. An even better complex systems scientist can borrow from other fields of research and using that knowledge to extend the model to include a new, more complicated assumption, which will make the whole process more complex. And that basically is a complex system.
Examples of Complex Systems
Not every extension of a model will make it directly a complex system: the problem itself needs to become fundamentally harder. To keep you some feeling of complex systems, I will describe three examples.
Independence assumption
Especially in statistics, and ‘identically independent distributed process’ is one of the favorite toys of scientists. The independency assumption basically means that when two things happen, they do not depend upon each other. Take for instance the waiting line at the supermarket: people are assumed to be joining at random. However, when the waiting line is very long, you might decide that you need to shop faster and join sooner (because you need to make an appointment), then when the line is short. So, the process may not be independent. What happens in the case of dependency is what Jim Crutchfield talked about today (all day, and still not finished ;) ).
Continuity assumption
Both in math and in physics, often it is assumed that you can approximate a discrete process by a continuous process. Some of you may remember the Riemann-sums (blocks underneath the graph) to approximate the area underneath the graph. The next step was to integrate the function, and use that to calculate the area underneath the graph. The latter (integrated function) is the continuous process version of the discrete process (the Riemann-sum).
Once you have integrated the function, calculating the area underneath a graph is easier than with the Riemann-sum – especially when you want to do more advanced stuff. That is why often people approximate a discrete process by a continuous process: as it generally makes things easier, and allows for exact mathematical results. But, a continuous process is not always a good representation of a discrete process.
A counter example turns out to be childishly simple (and fun). Remember the little water/sand mills for children? You put in a substance on the top, and as soon as the first bucket is full, the wheel starts to turn. If you have a continuous flow of e.g. water, the wheel will keep turning – and in this case a continuous process is a good approximation to find e.g. the speed of the wheel. But, when we take the same wheel, and we slowly start dripping water into it, something different happens. At some frequency (and you can try this yourself) the little bucket will drip full slowly, and then turn a bit as it loses balance. A new little bucket is up, and that will drip full – but may start turning the wheel in a different direction then the one before. Predicting which way the wheel will turn, becomes a very difficult task – which issue would never be clear from the continuous process. So, the discrete process shows a feature that we cannot capture in the continuous representation.
Off course, the way a child’s toy turns seems not vital to the working of the world at large. But in fact, this is a small model of how a discrete process can result in behavior that is different from the continuous approximation – and that discovery (and playing with that) is complexity science, too.
Networks
Finally, I couldn’t stop before I mentioned networks. In short, this is the field of studying interactions and relations between all kinds of things: people, the internet, power grids, and so on. Basically, the network is studied in terms of connectedness, robustness, distance between nodes, and much more. Secondly, they link these things to research fields in which networks are important, such as transportation, but also human interaction. In the spread of (contagious) disease, the network of people connecting to one another is a vital part of the way disease spreads. So, studying epidemic outbreaks as a complexity scientists, you would include the (social)networks of the people involved in finding the best way to fight the disease.
'New techniques'
Generally, complex systems are at the front end of their research field (or, on the far boarder of their fields, if you are a bit more skeptical). You need some guts when you decide to study the internet as an organism. You need to have a dream if you are working on building a super computer with far more power than the human brain. And you may be considered plainly stupid by some, if you spent your time programming little agents who interact with each other. In short, as a complexity scientist you need to be confident and believe that you will bring science to the next level.
These complex systems are complex because they cannot be easily solved by standard technology of the field, new methods need to be developed – or can be developed using complex systems. A good example of the latter is that Alfred Huebler can let small iron bits play tetris (but as mentioned before, Alfred Huebler is said to be able to do anything if he just ‘puts up the throughput’- including wrestling crocodiles). An example of the former (new methodology) is the ever growing attention for agent based modeling: in the computer program Netlogo this would be equivalent to programming how turtles interact with each other: basically making a ‘game’ in which you tell every single turtle to e.g. turn around when he sees land, and go towards the nearest turtle he can see. All of that is complex systems research.
Why should you be happy there are ‘complex systems scientists’?
In case you are a scientist yourself, this is pretty easy: complexity research is a lot of fun, you can do very different things, meet people from many different fields, it allows for an open mind, creativity and challenging problems – and on top of that, it is very much in fashion in the greater scientific community, which may benefit your chance of getting something published. Chances are, you are not a scientist. Why should you care? Computers taking over the world doesn’t sound like a good thing to invest taxes in, I could imagine. I am not going to completely contradict that notion: it is true that some of this research is pretty wild, and ‘we’ are not sure if ever anything useful will come out. I do not know if this is a consolation, but that is unfortunately true for a lot of research (if not all).
There is an upside: complexity research so far has lead to some pretty cool results! When trying to prevent an epidemic (large spread of disease) I think it makes sense to take into account that a busdriver will meet far more people that the average ‘I prefer to work at home’ scientist – and taking that into account in to the modelling may make the way we fight spread of disease far more effective. Another example I find pretty cool, is that someone has made an agent-based model of a long-gone cultural society. Archeologists are better able to reconstruct and explain/clarify the way the people lived. For instance, they find that after so many years food starts to get scars in the area, so the import chicken to keep near their houses, so that they have enough to eat.
The same agent based models can explain some of the stuff currently going on in our society: from segregation of ethnicities to marriage… So, although we are not sure all complexity research is of benefit to our world, there are some pretty neat examples that say complexity research makes the world a little bit a better place.
In summary
In essence, complexity goes back to the roots of the model and the problem, compares this to the world, finds an important feature that is still missing from the model, and tries to add this. Additions can be e.g. interactions between things in the model, nonlinearity (not everything is a straight line), dependency of things (not all processes are purely random), and so on. To solve these problems, complexity researchers try to borrow from many fields, but two of their favorites are statistics and mathematics. Another option instead of borrowing is to develop new techniques to study (new) problems.
Not all complexity research is immediately clearly usefull. Still, there are good examples of where complexity research has made a huge difference in the way we understand or solve things, such as understanding and accommodating for interactions in a process (think of the disease spread and network).
Complexity researchers come from all different kinds of backgrounds and fields – and this probably causes the fact that there is no single, clear definition of a complex system. It might even be (in my opinion), that the current ‘new’ assumptions of complexity will get accepted in each of their respective fields, and the in some years the current labeled ‘complex systems’ will not be considered to be complexity anymore, but continue their lives under a different name.
I hope though, that the essence of this complexity field will not fade. In this highly fractured world, I think it is so very important to try to come together with people from different fields and with different specialties, learn from each other and be able to step back and compare our research to what we are actually studying: the place we live. I think this is vital to do good research and especially try to push research forward in a more converging instead of diverging way.
Meeting different people and comparing your own believes and assumptions with the world, can be rewarding for everyone - scientist, and non scientist. Therefore I would like to end, now that you have a little bit of feeling for complexity, by wishing everyone a little complexity in their life.